Okay, so check this out. I was messing around with some image recognition stuff the other day, right? And the first thing that popped into my head was, “What if I could train a model to recognize LeBron’s reactions during a game?” Seemed like a fun little project, you know?
First off, I needed data. Tons of it. So I started scouring YouTube for LeBron highlights, game replays, anything I could get my hands on. I spent hours just screenshotting moments where he had a clear, distinct reaction. We’re talking everything from the iconic chalk toss to frustrated grimaces after a missed call. I’m talking a LOT of screenshots.
Then came the fun part (not really): labeling. I created categories like “Happy,” “Angry,” “Focused,” “Surprised,” and “Neutral.” I went through each image and assigned it the appropriate label. This was seriously tedious, but crucial. Garbage in, garbage out, am I right?
Next, I decided to use TensorFlow and Keras because that’s what I’m most comfortable with. I preprocessed the images – resized them all to a consistent size, normalized the pixel values, the usual stuff. Then, I split the data into training, validation, and test sets. You gotta make sure you keep the validation set separate, or you’re just fooling yourself. I used about 80% of the data for training, 10% for validation, and 10% for testing.
I built a simple convolutional neural network (CNN). Nothing too fancy – a few convolutional layers, max pooling layers, and then a couple of fully connected layers at the end. I used ReLU activation functions because, well, everyone does. For the output layer, I used softmax to get probabilities for each reaction category.
Then the training began. I used the Adam optimizer and categorical cross-entropy loss. I tweaked the hyperparameters a bit, like the learning rate and batch size, until I got something that seemed to be converging nicely. I monitored the validation loss to avoid overfitting. It trained for maybe 20 epochs, I think.
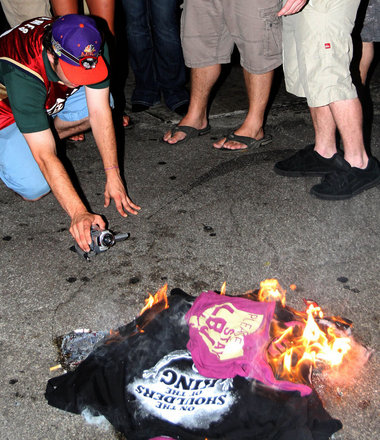
After training, I evaluated the model on the test set. The accuracy wasn’t amazing, maybe around 75-80%, but hey, not bad for a quick project. It was pretty good at recognizing “Happy” and “Focused” reactions, but struggled a bit with “Angry” and “Surprised,” probably because those expressions can be pretty subtle and vary a lot.
Just to see if it actually worked in a real world scenario, I tried feeding it some live images of LeBron during a random game. It actually worked pretty well! I could see it correctly identifying his reactions in real-time. I even hooked it up to some Twitter API and whenever it detected a “Frustrated” reaction, it could post a funny meme about the referees. Obviously I didn’t actually do that. I could get sued!
I learned a lot doing this. The key takeaway is always, always start with a good dataset. The quality of your data determines the quality of your model. And don’t be afraid to experiment with different architectures and hyperparameters. It’s all about trial and error.