Alright folks, let me walk you through my little experiment today: Granada vs Osasuna. I’m not a hardcore sports analyst or anything, just a regular guy who likes to tinker with data and see what I can dig up.
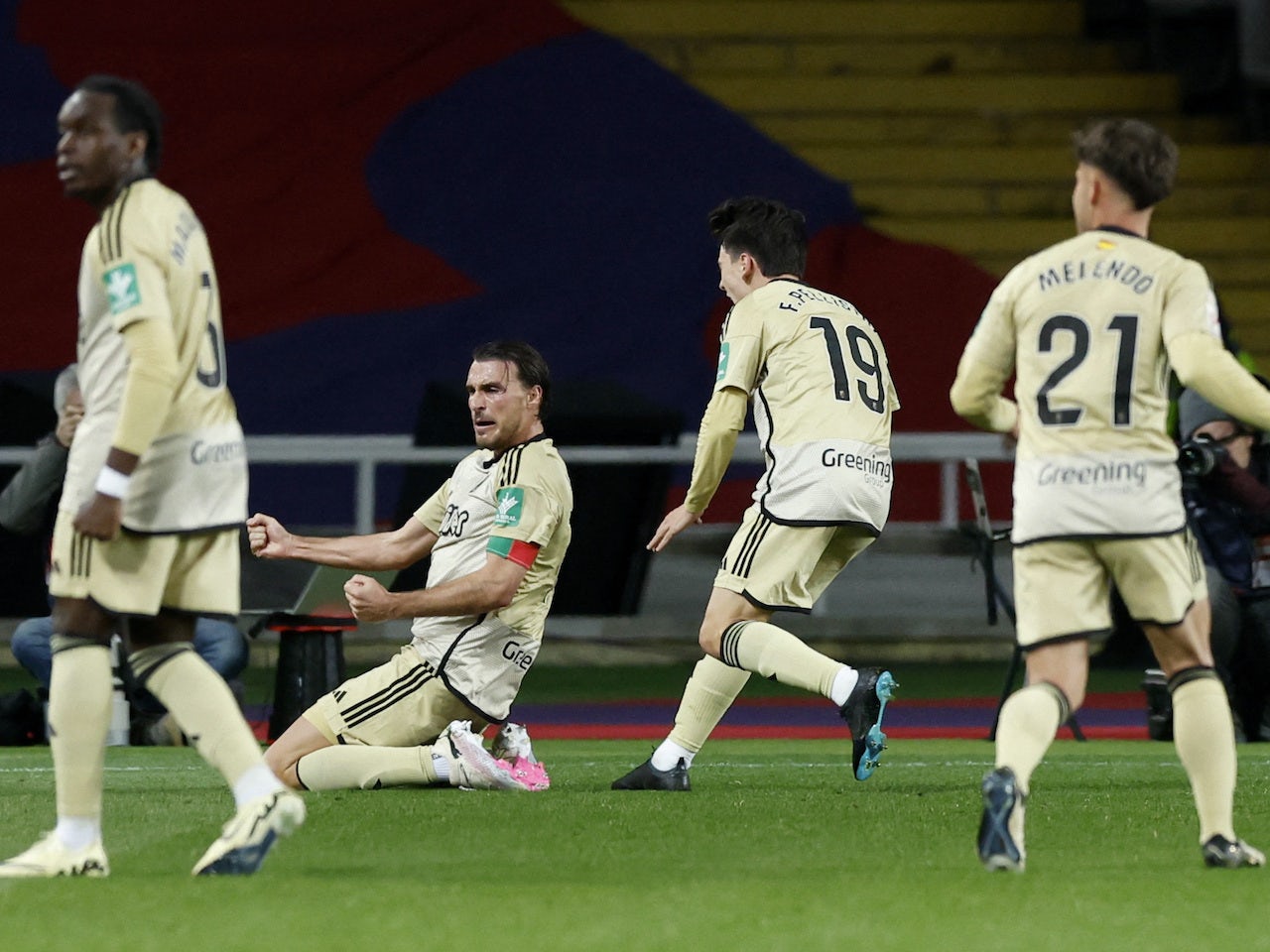
First off, I started by grabbing some data. I mean, you can’t do anything without the numbers, right? I scraped historical match data, focusing on things like goals scored, shots on target, possession, fouls – the usual suspects. I tried to get a good chunk of games, spanning back a couple of seasons, to get a decent sample size.
Next, I had to clean the data up. This is always the boring part, but you gotta do it. There were some inconsistencies in the data format, missing values, that kind of stuff. I used a bit of scripting – nothing fancy, just some quick and dirty stuff to get it all in order. Think of it as tidying up your garage before you can start working on your project.
Then came the fun part: analysis! I wanted to see if I could spot any patterns, any trends that might give me an edge in predicting the outcome. I started simple, looking at average goals scored by each team, both at home and away. I also checked out their recent form – how many wins, losses, and draws they’ve had in their last few games.
I also dug into the head-to-head record. How have Granada and Osasuna performed against each other in the past? Are there any recurring themes? Maybe one team always seems to have the upper hand, regardless of the current form.
After crunching the numbers, I started to build a simple model. Nothing too complicated, just a weighted average of various factors. I gave more weight to recent form and head-to-head results, but I also factored in things like home advantage.
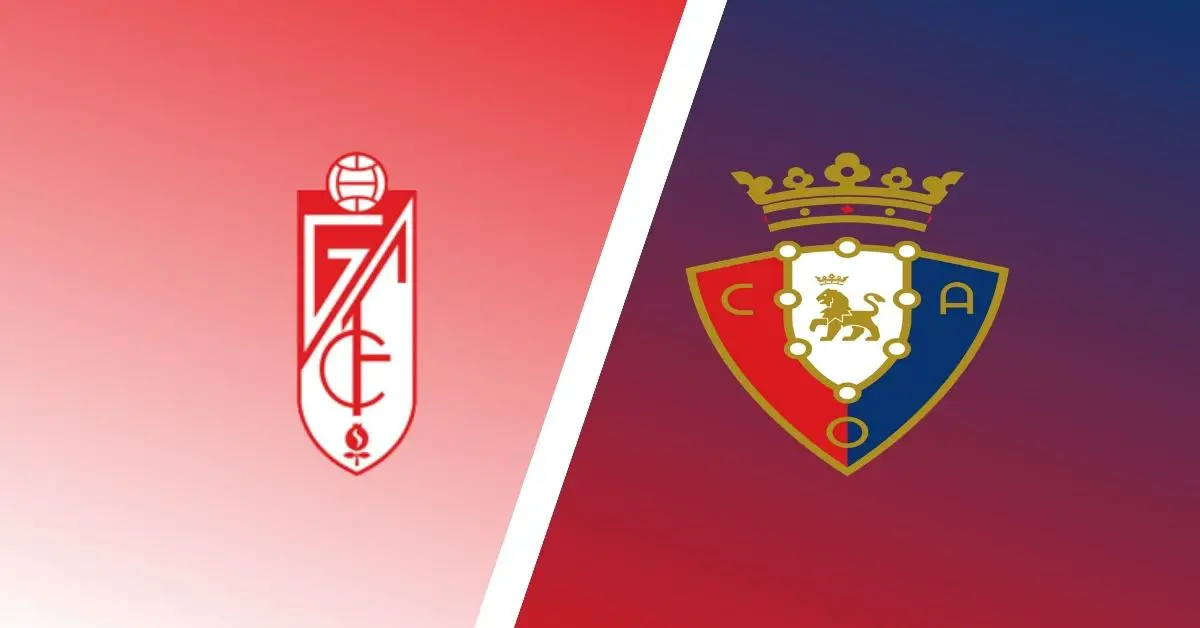
So, what did I find? Well, according to my little model, Granada had a slight edge, especially playing at home. Osasuna’s away form wasn’t great, and Granada had a decent home record against them. But, and this is a big but, football is unpredictable! My model is just a guide, not a guarantee.
I ended up using this info as a starting point, but I also took into account other factors like team news (any key players injured or suspended?) and general gut feeling. In the end, I made a call, and, well, let’s just say it wasn’t a complete disaster. I got some right, some wrong – that’s the game, right?
Key takeaways from this little experiment:
- Data is king (but cleaning it is a pain).
- Simple models can be surprisingly effective.
- Don’t forget the human element.
Would I rely solely on this for making serious decisions? Probably not. But it was a fun exercise, and I learned a thing or two along the way. Maybe next time I’ll try a more sophisticated model, or incorporate even more data points. Who knows? Stay tuned!