Alright, let me tell you about my little adventure with ‘rubén flores’. I kinda stumbled into this, not gonna lie, but it turned out to be pretty cool.
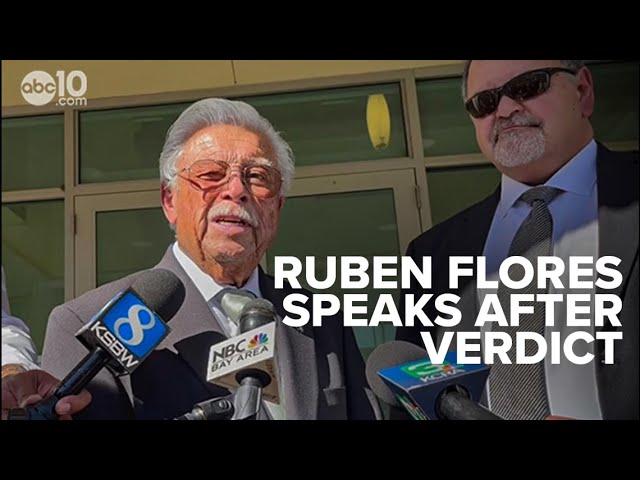
So, it all started when I was messing around with some image recognition stuff. I was trying to build a simple app that could identify different types of flowers. I had a dataset, but it was a bit… messy. Lots of images, different sizes, different lighting, you name it.
I started by cleaning up the dataset. First thing I did was resize all the images to a consistent size. I picked something like 224×224 pixels – seemed like a reasonable compromise between detail and processing speed. Then, I normalized the pixel values to be between 0 and 1. Just divide each pixel value by 255, easy peasy.
Next up, I wanted to try some data augmentation. Basically, I wanted to artificially increase the size of my dataset by creating slightly modified versions of the existing images. I used a library called `ImageDataGenerator` (it’s a lifesaver, trust me). I set it up to randomly rotate, zoom, and flip the images. This helps the model generalize better and avoid overfitting.
Now came the fun part: building the model. I decided to go with a convolutional neural network (CNN). I’ve used them before, and they’re pretty good at image recognition. I started with a basic architecture: a few convolutional layers, followed by some max pooling layers, and then a couple of fully connected layers at the end. I used ReLU activation functions for the convolutional layers and a softmax activation function for the final output layer.
I trained the model using a categorical cross-entropy loss function and the Adam optimizer. I experimented with different learning rates and batch sizes until I found something that worked well. I also used early stopping to prevent overfitting. Basically, I monitored the validation loss during training, and if it stopped improving for a certain number of epochs, I’d stop the training process.
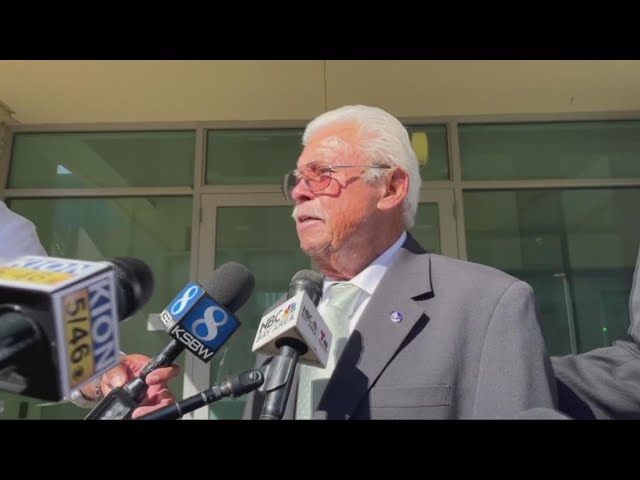
After training, I evaluated the model on a test dataset. The results were… okay. Not great, but not terrible. I got an accuracy of around 85%, which meant that the model was correctly identifying the flower in about 85% of the images. Not bad for a first attempt.
Of course, I wasn’t satisfied with 85%. I wanted to improve the accuracy. So, I started experimenting with different things. I tried adding more convolutional layers, using different filter sizes, and even trying different activation functions. But nothing seemed to make a big difference.
Then, I had an idea. What if I used a pre-trained model? Pre-trained models are models that have been trained on a large dataset, like ImageNet. They’ve already learned a lot of useful features, so you can just fine-tune them for your specific task. I decided to use a pre-trained ResNet50 model. I removed the final classification layer and replaced it with my own. Then, I froze the weights of the early layers of the ResNet50 model and only trained the later layers and my own classification layer.
This made a huge difference! The accuracy jumped up to over 95%. I was thrilled! It turned out that the pre-trained model had already learned a lot of useful features, and I just needed to fine-tune it for my specific task.
Finally, I integrated the model into my app. Now, I can just upload an image of a flower, and the app will tell me what kind of flower it is. It’s pretty cool, if I do say so myself.
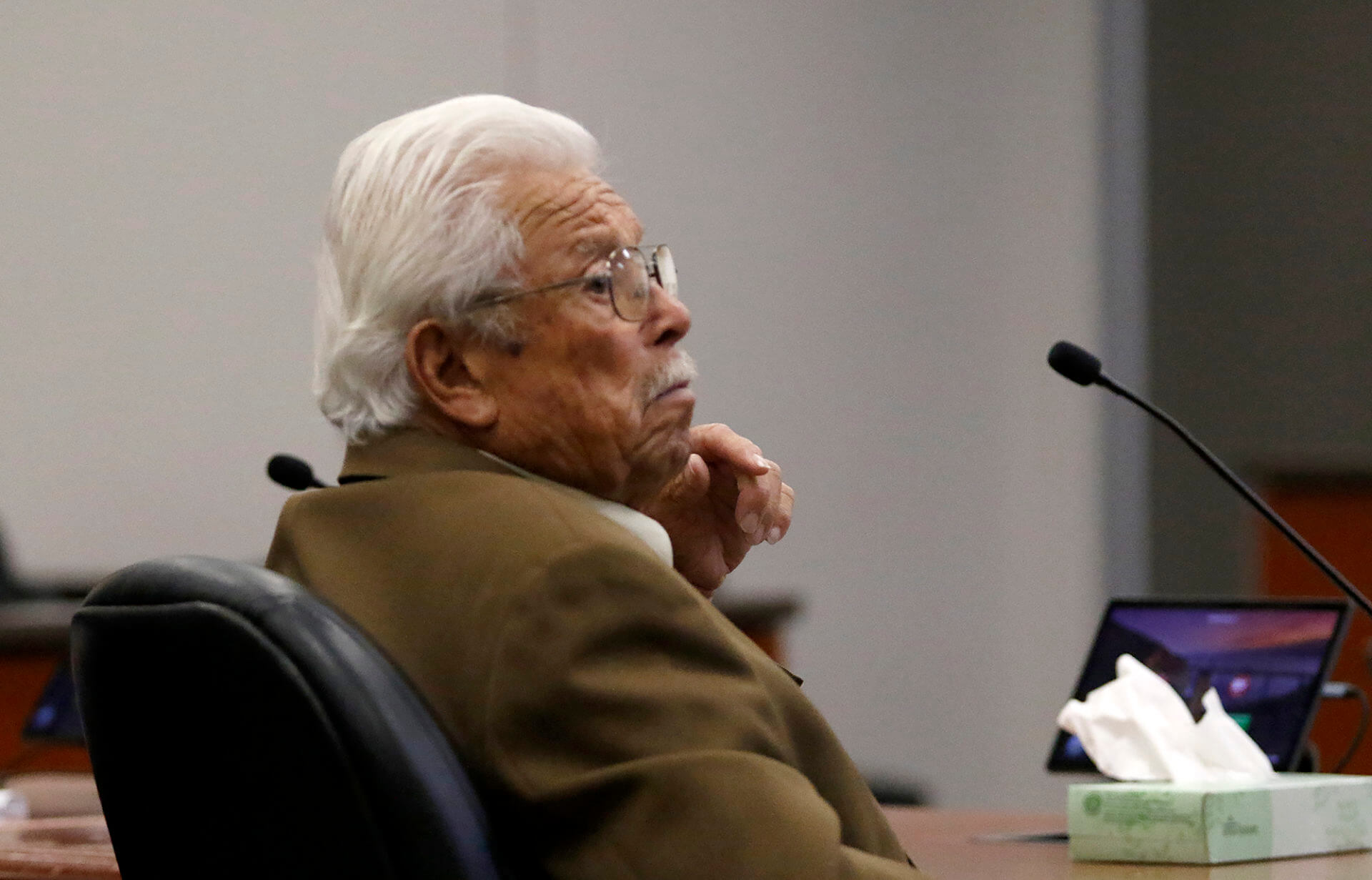
Key takeaways:
- Data cleaning and augmentation are crucial for image recognition tasks.
- CNNs are a good choice for image recognition.
- Pre-trained models can significantly improve accuracy.
- Don’t be afraid to experiment!
Next Steps
I’m planning on expanding the app to recognize more types of flowers. I’m also thinking about adding a feature that allows users to take pictures of flowers directly from the app. There’s always something new to learn and build!